How Happy is Your Virtual Brain? The Exciting Intersection of Digital Twins and Large Language Models
Entering your psyche with large language models
In this edition:
Digital twins (DT) are useful as virtual representations of patients (or their organs) to test therapy and improve disease understanding.
Large Language Models (LLM) can complement traditional “biophysics-based” DTs by offering a way to personalize through natural language in a setting where disease mechanisms are not easily captured by exact models.
For example: a virtual brain, combining DT and LLM technology, could help doctors and patients understand mental states, provide reflection tools, and perform virtual therapy.
Combining DTs with LLMs could create a new field of research and make healthcare more effective, accessible, and personalized.
Read time: 3 minutes.
Welcome to this new edition of Transforming Med.Tech, the newsletter in which I try to understand how disruptive innovations will transform medical technology. In this edition, I’m diving into the overlap between exact models of biophysics and the fuzzy reasoning of chatbots.
It can be very useful to have an exact duplicate of an object: a physical twin. During the Apollo 13 mission, the astronauts were stuck in the lunar module on their way back to Earth after a disaster. The module's carbon dioxide removal system wasn't equipped for this situation and it was crucial to modify it to handle the increased usage. The engineers on Earth devised a solution using parts that they knew were available on the Apollo 13; they used physical twins. The astronauts were given instructions to implement this fix, which helped them survive.
Revolutionizing personalized medicine through virtual patient models
Now, let's dive into the world of digital twins, the virtual version of such representations. In healthcare, a digital twin of a patient can be a virtual representation of a patient's organ (or complete patient, although still utopian). It can be personalized to include the patient's particular characteristics and then used for testing therapies or improving our understanding of disease. An example of this approach is to use a scan of a patient's heart and combine it with our understanding of the role that scars play in infarcted hearts, to help predict if the patient has an increased risk for arrhythmias and may benefit from invasive ablation therapy.
These digital twins typically focus on modeling biological processes and diseases, and allow for virtual interventions to test what would be most helpful for that particular individual. Digital Twins (DT) have gained popularity, especially in the field of cardiology, where we have a relatively good understanding of the biophysical processes of diseases and thus can model them into a DT.
Thus, essentially DTs help to move from our “traditional knowledge,” which comes from medical trials and thus yields insights at cohort-level…
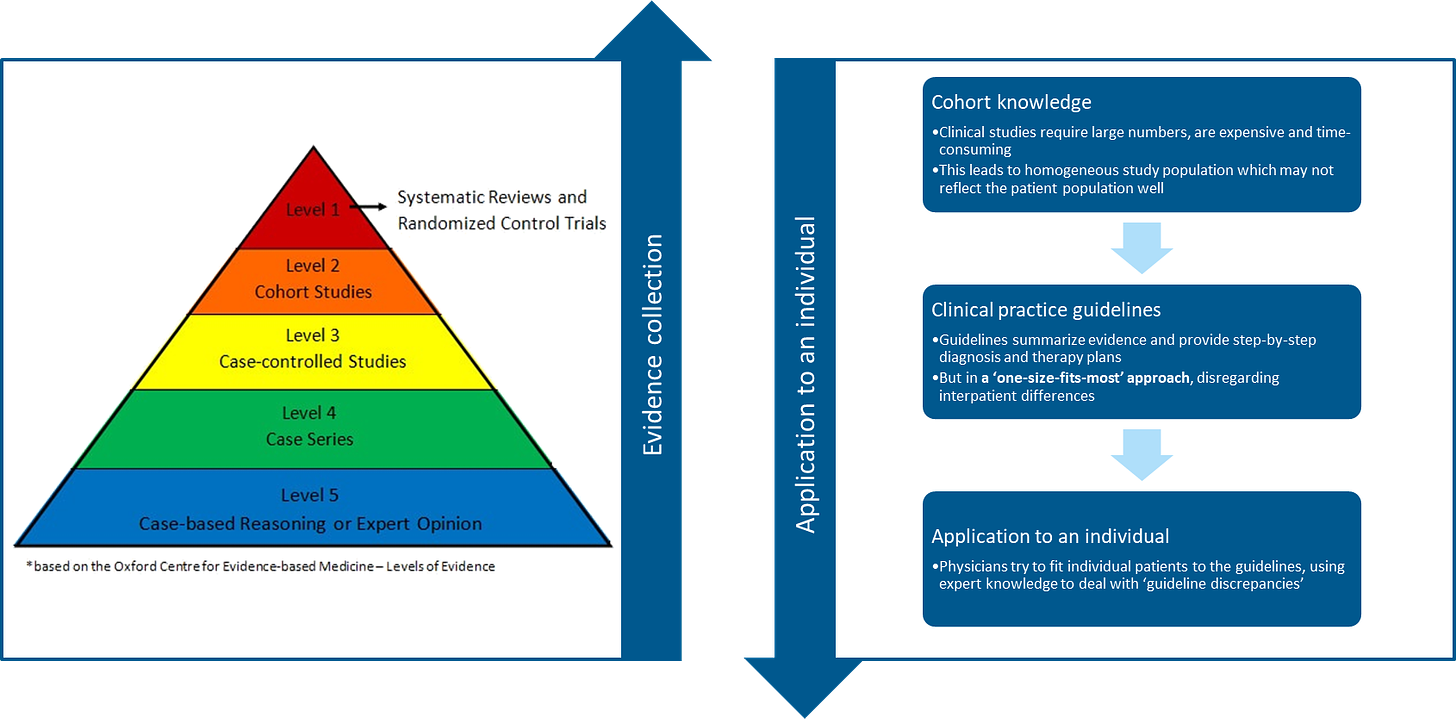
... towards more robust application of disease knowledge to a particular individual:
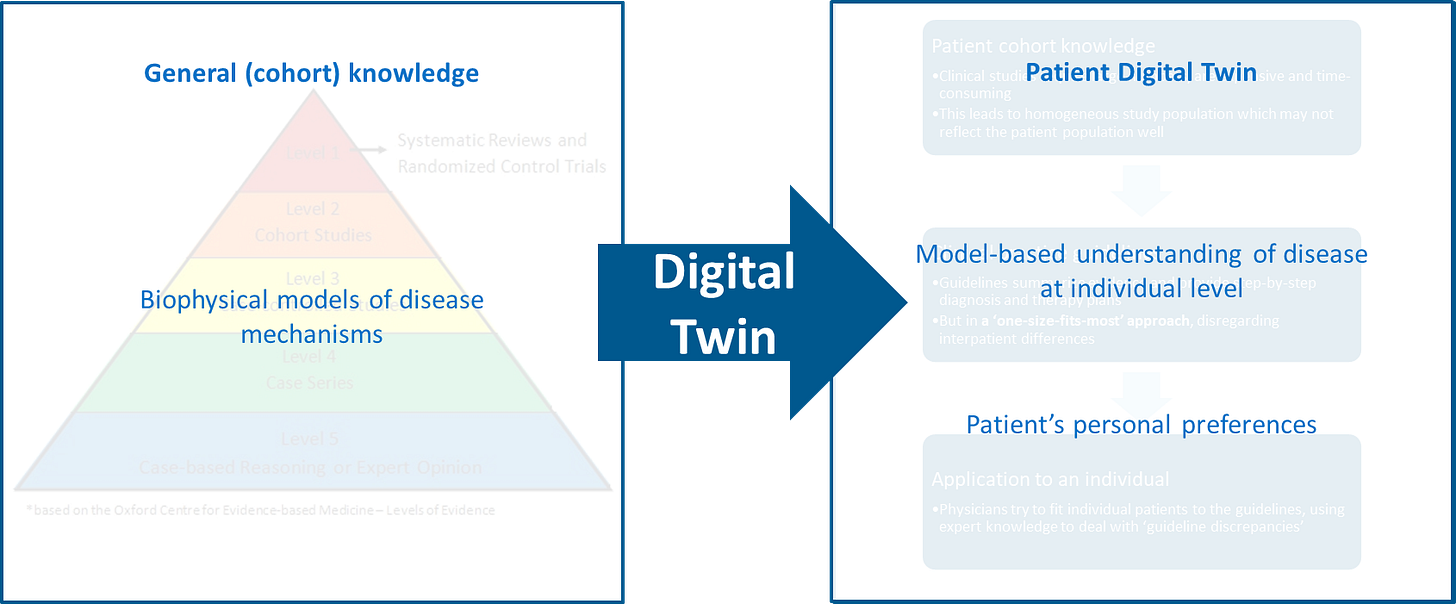
The critical aspect here is the combination of disease understanding and its personalization to that individual. I've worked in this field for years now and although clinical adoption is still challenging, there is a huge potential for personalized medicine if we can optimize the process of DT creation.
Blending Digital Twins and Large Language Models for personalized mental health care
Now, let's bring Large Language Models (LLM) into the mix. An LLM is a statistical method that predicts the next word in a sequence of words, and is typically trained on massive datasets of text. GPTx (by OpenAI, and used in Microsoft's Bing) and PaLMx (used in Google's Bard) are the most notable examples. Integrating LLMs with digital twin technology could open up potential applications in fields where we do not (yet) have exact biophysics models of disease.
For instance, Dan Shipper wrote an insightful article about using an LLM to take a personality test for him. He trained an LLM on his personality by feeding it personal data, such as daily notes and psychological assessments. Interestingly, when answering questions about his personality, the LLM performed better than his girlfriend and his mother. 🤯
Imagine creating a DT of a patient’s “psyche” by training an LLM on a patient's medical records: an LLM-DT. This LLM-DT could help both the doctor and the patient understand the patient's mental state more thoroughly. By asking probing questions similar to those a psychiatric professional would ask, the LLM-DT could serve as a reflection tool for the patient and help them understand their personality traits, strengths, and weaknesses. This could provide mental support to individuals who do not currently have access to it, promoting equality and democratizing healthcare access. It would also reduce the workload of the overburdened psychiatric staff.
But here is where things get exciting: the LLM-DT could be used to determine which specific personality traits or psychological challenges would benefit most from treatment for that individual. For example, we could tell the LLM-DT to reflect the patient, but be more open to new things (version A of the LLM-DT) or more impulse-controlling (version B). We could then again ask the “treated” LLM-DTs questions to determine how they score, for example on a general happiness metric. By tracking the outcomes of the patient's LLM-DTs after applying virtual therapy, they could help both the doctor and patient better understand the potential effects of therapy, which vary from person to person. You could use it to virtually figure out the best therapy to improve your mental health.
This reasoning may also apply to other fields where our understanding of disease is less well captured by exact biophysical processes. And since natural language is present throughout our lives, it may be one of the most valuable ways to create DTs that accurately represent not just our mental state, but also our norms, values and preferences. This could help to further personalize medical therapy.
In conclusion, merging digital twin technology with large language models could give rise to an entirely new field of research and transform healthcare as we know it. The combination of these technologies heralds an exciting era for personalized medicine, making healthcare more effective, accessible, and tailored for each individual.