Raising Kids and AI: Fostering a Deeper Understanding of Reality
Reflections on Raising a Digital Native and the Evolution of AI in Medicine
The highlights:
Shifting from unimodal to multimodal learning in AI is essential for a more complete world perspective
Multimodal medical models will have an increased disease and therapy understanding
Specialist and generalist AI may balance and correct each other
Read time: 7 minutes
In this edition of Transforming Med.Tech, I am diving into the fascinating parallels between raising a kid and training an AI, and what a more complete world perspective would mean for AI operating in healthcare.
First tech experiences as a kid can be transformative
As I am raising my first kid (and about to welcome our second), I'm reminded of the transformative power of technology, impacting my curiosity as a kid. One of my earliest memories of the internet is watching pixelated images of Mars sent from a lander unfold on my father's computer. I was exhilarated by seeing otherwordly images mere hours after they had been captured on a planet so far away. I was similarly excited by the shift in knowledge access, from the impressive printed volumes of Encyclopædia Britannica at my grandparents' bookshelf to a CD version that you could search in, easily exploring new concepts, later only to be made even more accessible by free online resources such as Wikipedia.
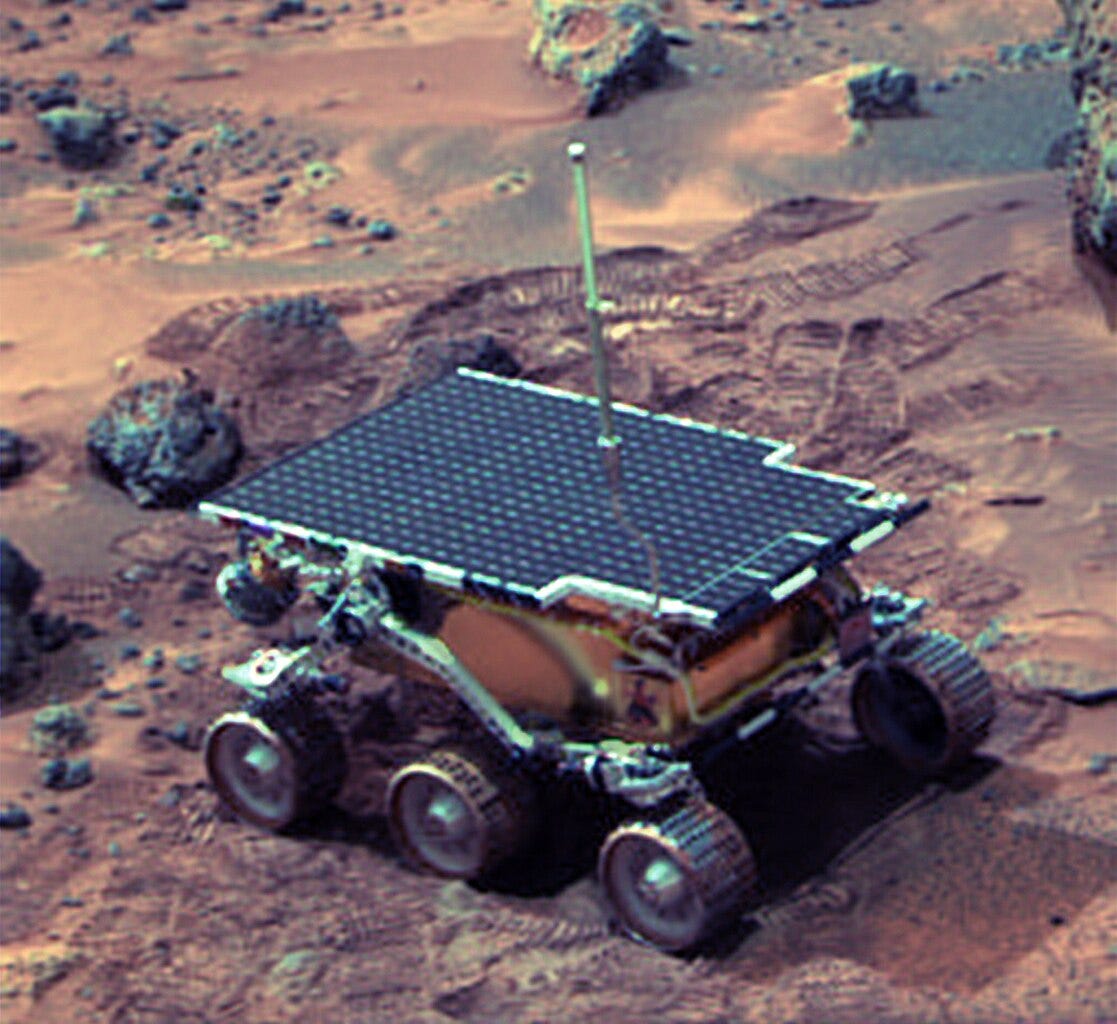
In contrast, my two-year-old is already used to much more advanced technology, asking our smart speaker to play music when she wants to dance. She'll be an expert in using AI in another year or so, benefiting from a chatbot's large language model (LLM) to explain concepts with words she can understand. Whereas I am sometimes starting to feel old when I use tech (who keeps redesigning all those perfectly fine UIs...), she'll grow up to have enhanced tech versatility. (Of course, one can wonder whether that's a good thing... Shouldn't she learn to think for herself? It's that century-old question that we have asked ourselves when books/newspapers/radio/tv/internet/smartphones came out...)
We need to transcend text to really understand the world
Raising a kid also makes you think about learning. As a baby, you gradually grasp more and more concepts, bringing together experiences from sight, sound, touch, and feelings. On the contrary, LLMs are trained on a text-only corpus. And while LLMs are not explicitly trained to reason, the size of their neural networks and training data has resulted in 'emergent behavior' that, from an outside perspective, mimics reasoning. Restricting LLMs to unimodal (text-only) sources will limit that emergent behavior. Therefore, research is diving into multimodal foundation models. Such models learn not only from text but also from images, video, sound, etc. They have even strapped a camera on baby Sam for 19 months to see if this 'natural' data collection would work, too.
Multimodality is critical to obtaining a more complete 'world image' and getting a valid (or at least better) understanding of how the world works. Imagine learning only from text, never seeing schematics that create that 'aha' insight, hearing the emotion in a person's voice, experiencing the wind on your skin or the smell after rain. A unimodal foundation model simply cannot 'imagine' the full range of those experiences, like flatlanders in a 2D world cannot imagine the third dimension.
Do apples always fall?
The most recent advancement towards a more complete 'world image' is evident in OpenAI's Sora, which allows the creation of minute-long videos from a text prompt. Amazingly, the videos (mostly) follow the laws of physics without being told how physics works.
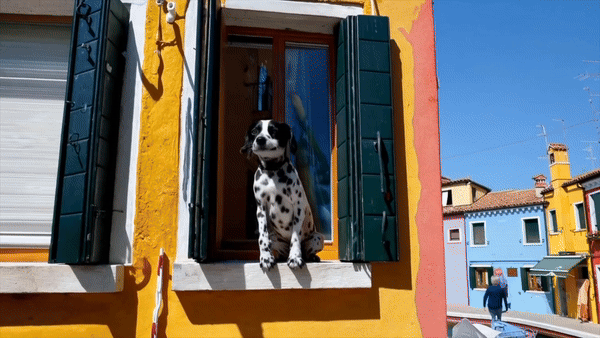
Whereas game and animation engines have dedicated physics simulators, text-to-video foundation models can now work without such explicit knowledge incorporated. This hints that AI's worldview is becoming more complete. Perhaps it does not truly understand physics: it may only be repeating common patterns from its training data, which probably does not include physics-defying levitation. Still, the similarities with how a kid learns are apparent: my kid has never seen a levitating apple either and would be utterly surprised if she did. So although my daughter doesn't have a full understanding of physics yet either, she is capable of grasping the basic concept (even if she, literally, stumbles occasionally).
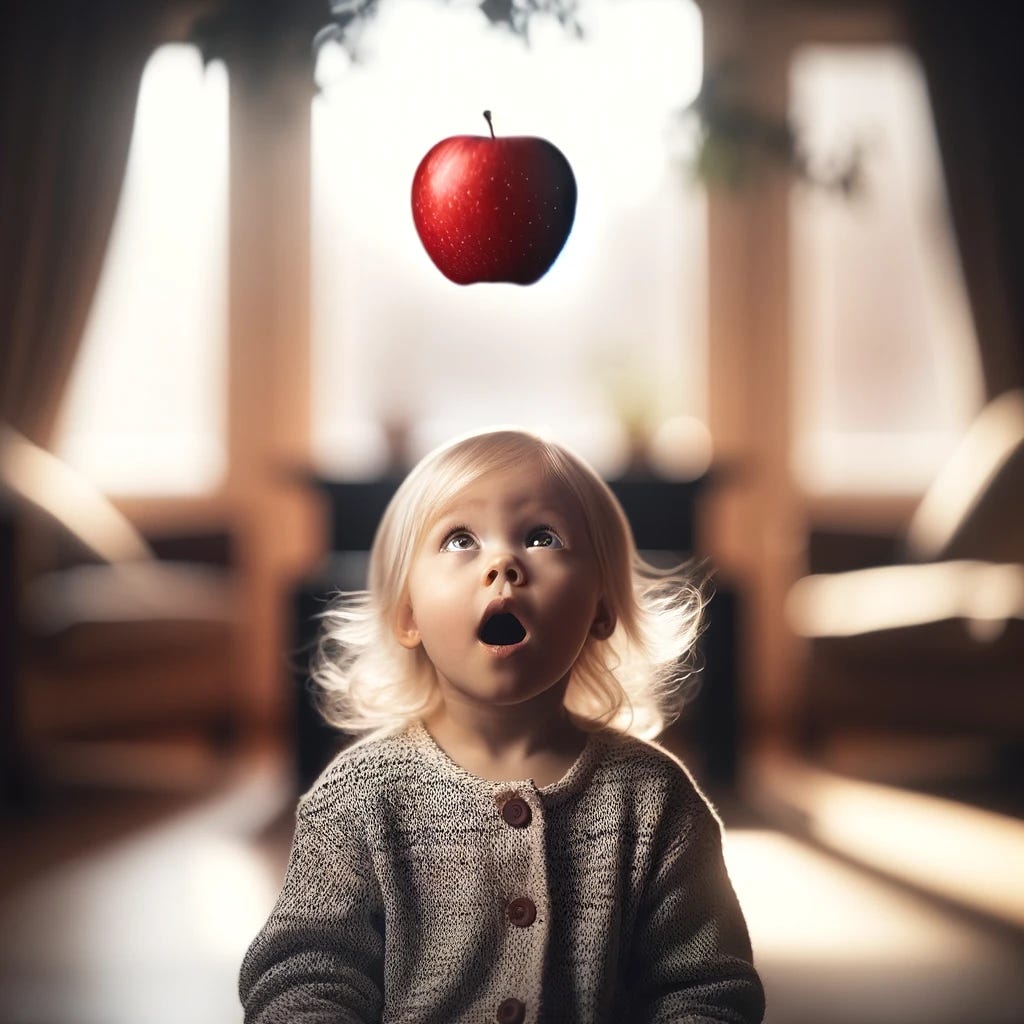
What multimodality means for healthcare
How does this translate to healthcare? In medicine, the move towards multimodal models would signify a leap towards more holistic and nuanced medical knowledge. An LLM can already read medical guidelines and use text to apply them to your patients' medical records. But if this is fully text-based, its 'knowledge' of the disease is also purely textual. During my studies, I experienced that illustrative schematics or hands-on time with patients provided invaluable insights in addition to text-based learning. A medical world image is more complete if it transcends text and includes a feel for three-dimensional anatomy, images, processes, physiology, diagnosis, and therapy. Some work is already being performed in this area: in the imaging domain, models can quite literally transform from one imaging modality to another, and multimodal prediction models outperform unimodal models in predicting drug response. As an arrhythmia scientist, I would love to see a foundation model trained on the heart from a 3D anatomy, electrophysiology, cellular, organ-level, nervous innervation, and physics perspective, helping me interpret imaging, electrocardiograms, phonograms, drug responses, questionnaires, and other multimodal patient data.
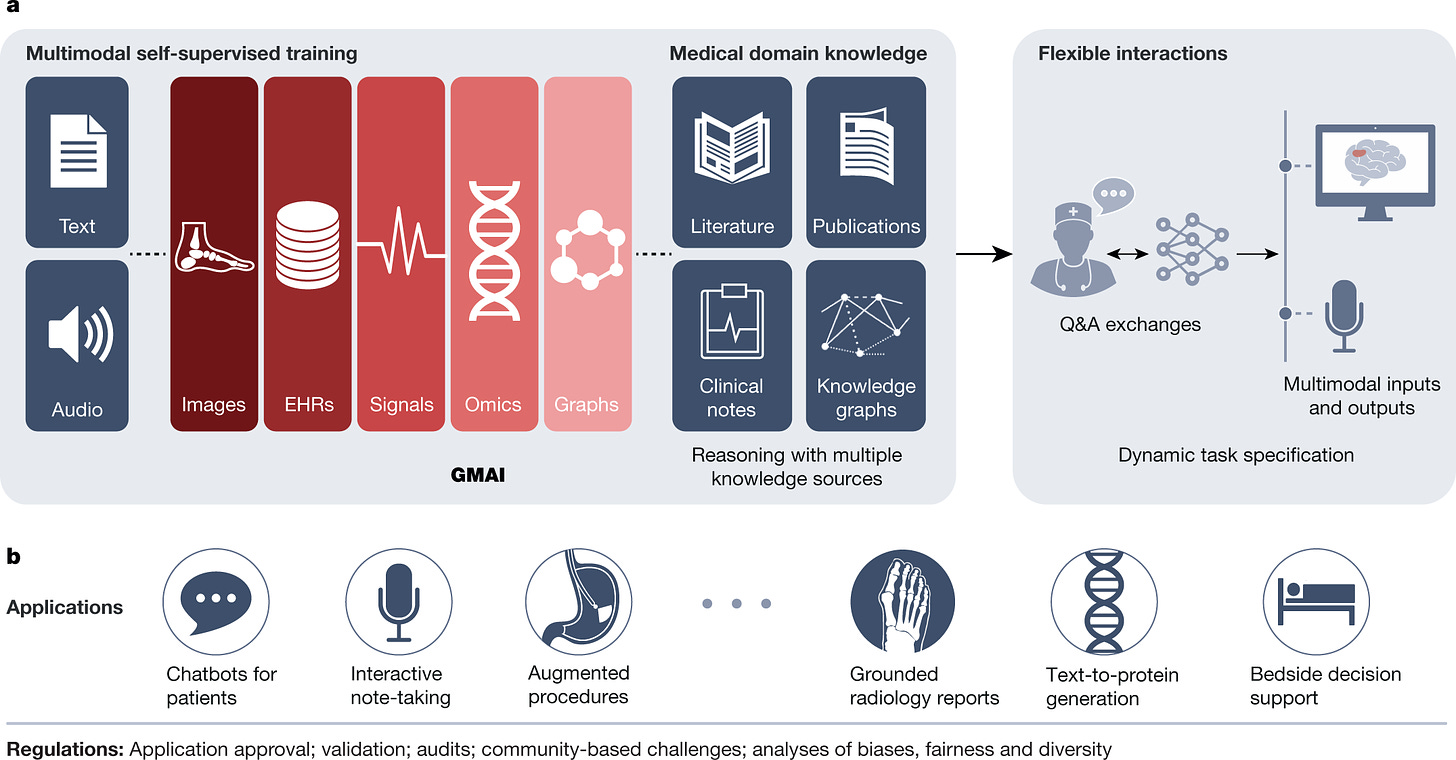
Do we need specialist or generalist AI?
However, we aren't there yet. In the near future, we'll be working with highly-specialized unimodal models, and moving to more encompassing 'large medical models' will take some time. This is similar to how we developed as a species: first we got specialists (farmers, rulers, mathematicians), which then allowed our society as a whole to obtain a better generalized world perspective. Ultimately, we will arrive at 'large medical models' or even 'large anything models,' but it may require another paradigm shift to reason from concepts rather than words, just like the transformer-paradigm has driven the current foundation model boom.
The specialist-vs-generalist perspective for AI, of course, also mimics our health care system, where a highly-focused specialist may provide the state-of-the-art treatment for a specific disease, and a general practitioner is immensely valuable in taking a holistic perspective and caring for the patient as a whole. Besides this essential harmony, there is another reason to strive for coexisting generalist and specialist AIs. LLMs are better at math that involves numbers that appear frequently in their training data (another sign that they do not internalize reasoning!). The focused and potentially biased attention of a specialist AI can be balanced by a more comprehensive perspective from a generalist AI. In general, fact-checking or safeguarding an AI with other AIs has been shown to improve their output quality.
Conclusion
The parallels between raising a child and training AI offer insights into the nature of learning, growth, and the pursuit of a comprehensive world image. Abstraction involves formulating general laws, beyond pattern recognition. Just like my kid is still learning that, current AI is too. This evolving understanding in AI not only mirrors human cognitive development but also promises to revolutionize healthcare, bringing forth innovations in diagnosis, treatment, and patient care that were previously unimagined. And as I embark on the journey of fatherhood once again, I am reminded of the endless possibilities that lie in nurturing the next generation—both human and artificial.
Hi Matthijs, great column. I wonder if LLMs as they are conceived now can ever get to what you propose based only on the simple statistical models that drive them. I mean, it is easy to imagine scanning text and looking for word-order patterns, without any need to understand meaning. But what connections can we imagine that would link images, diagrams, 3D models, etc? To me, text-based LLMs have to incorporate meaning before we can ever hope to go to higher dimensionality.
Cheers, Rob